AI in the pharmaceutical industry promises cheaper, faster, better drugs
Finding potential new drugs is becoming faster and cheaper, thanks to artificial intelligence, but challenges remain.
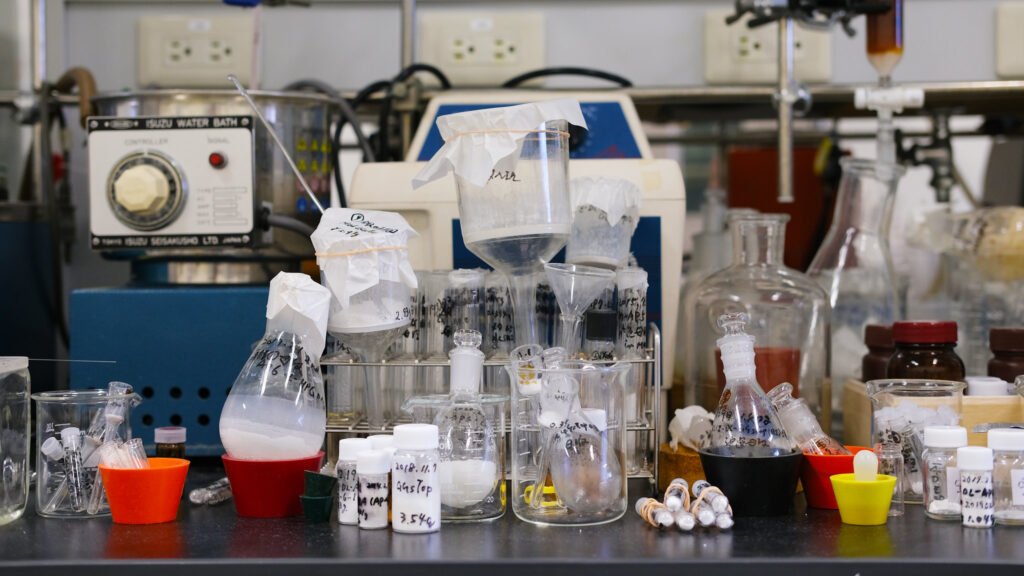
Finding potential new drugs is becoming faster and cheaper, thanks to artificial intelligence, but challenges remain.
AI was the hottest ticket in town for predictions during the pandemic. Highly sensitive and specific in identifying objects, quick to summarise information, and consistent in producing results, it seemed to be a panacea for our medical research troubles. However, COVID-19 also exposed the limitations of modelling. Computer models for virus spread are either very complex or, conversely, simplified to be practical on available computers.
The truth, as ever, is somewhere in the middle: while it’s not a solution in itself, AI can assist in diagnosis, treatment, prediction, and drug and treatment discovery, and can increase human ability to fight this and future pandemics.
Long before AI technology evolved, drug discovery and development was the work of medicinal chemists working together in a laboratory, testing and validating their syntheses. The process was long, expensive and slow; estimates are US$2.6 billion and 10 years on average for a new drug.
The emergence of artificial intelligence (AI), both machine learning (ML) and deep learning (DL), have helped accelerate the drug discovery and development process. The massive biological datasets around the world have become the raw material for drug manufacturing processing with an ML/DL-based approach. ML/DL can identify biologically active molecules with less time, effort, cost and more effectively.
Drug discovery requires a long and complex process which can be broadly divided into three main stages: object selection; compound screening; preclinical studies and clinical trials.
Those stages must be able to be transcribed and tested in an AI-based intelligent computing systems. If the drug candidate passes the safety phase and the efficacy has been confirmed in the clinical phase, then the compound is reviewed by agencies such as the United States Federal and Drug Administration (FDA) for approval and commercialisation.
AI-based drug discovery generally involves the computer in the first two of those stages, through drug design; automated synthesis; or drug screening – predictions on its bioactivity, toxicity, or chemical properties. Most diseases are associated with dysfunction of proteins in the body. The three-dimensional structure of proteins is hugely important and it is here that computer-assisted techniques can play an important role in the simulation and evaluation of protein structures.
Neural network-based algorithms to synthesise drug component molecules is expected to help scientists avoid failure and predict bad reactions.
Meanwhile, virtual drug screening is an advantageous computational approach to screen for molecules containing inappropriate ingredients in the early stages of drug development and efficiently find new hits.
But artificial intelligence faces some significant challenges, such as data diversity and uncertainty. The datasets available for drug development and discovery can involve millions of compounds. Traditional machine learning approaches may not be able to handle this amount of data. Deep learning with its neural network is considered a model that is much more sensitive to the prediction of complex biological or medical properties on random and huge time-series data sets.
However, the intelligent computational models also face the problem of experimental data errors when performing training sets and lack of experimental validation. That’s why, in some recent trends, many experts around the world are trying to develop adaptive learning approaches and hybrid methods that are enhanced by big data analytics. Several aspects of the drug discovery process have not been well explored.
Drug manufacturing requires close observations of the binding between potential drug molecules and their target proteins. Often it’s a challenging matter because the amount and quality of data to feed into the AI model may sometimes be insufficient. Sometimes a compound is tested using different methods which can produce completely different results, upsetting the algorithms.
Consequently, before carrying out AI-based approaches, filtering input from raw data is an essential step to obtain high-quality data.
AI technologies, especially DL algorithms, have proven to be of great support in the development and discovery of promising drugs in the big data era. DL is able to extract key features from large and massive pharmaceutical datasets. In addition, DL-based intelligent computing techniques can handle complex problems without human interference.
The sophistication of computerisation and brilliant synthesis technology in development and drug discovery could turn the impossible into the possible to bring solutions to society at low prices, small failure rates, and short cycles in drug development.
Integrating ML, DL, and human skills and experience from government, the private sector, universities, and the community can lead to pharmaceutical strength and medical resilience.
Feby Artwodini Muqtadiroh is a doctoral candidate at the Department of Electrical Engineering, Faculty of Intelligent Electrical and Informatics Technology, Institut Teknologi Sepuluh Nopember, Surabaya, Indonesia
Mauridhi Hery Purnomo is a senior researcher at the Department of Computer Engineering, Faculty of Intelligent Electrical and Informatics Technology, Institut Teknologi Sepuluh Nopember, Surabaya, Indonesia. He is the Chair of the Laboratory of Multimedia Computing and Machine Intelligence
I Ketut Eddy Purnama is a dean of Faculty of Intelligent Electrical and Informatics Technology, Institut Teknologi Sepuluh Nopember, Surabaya, Indonesia
The authors declare no conflict of interest.
Originally published under Creative Commons by 360info™.