We’d all love to have a smart robot in our house that understands spoken natural language, just like those in sci-fi films. How far are we from this dream?
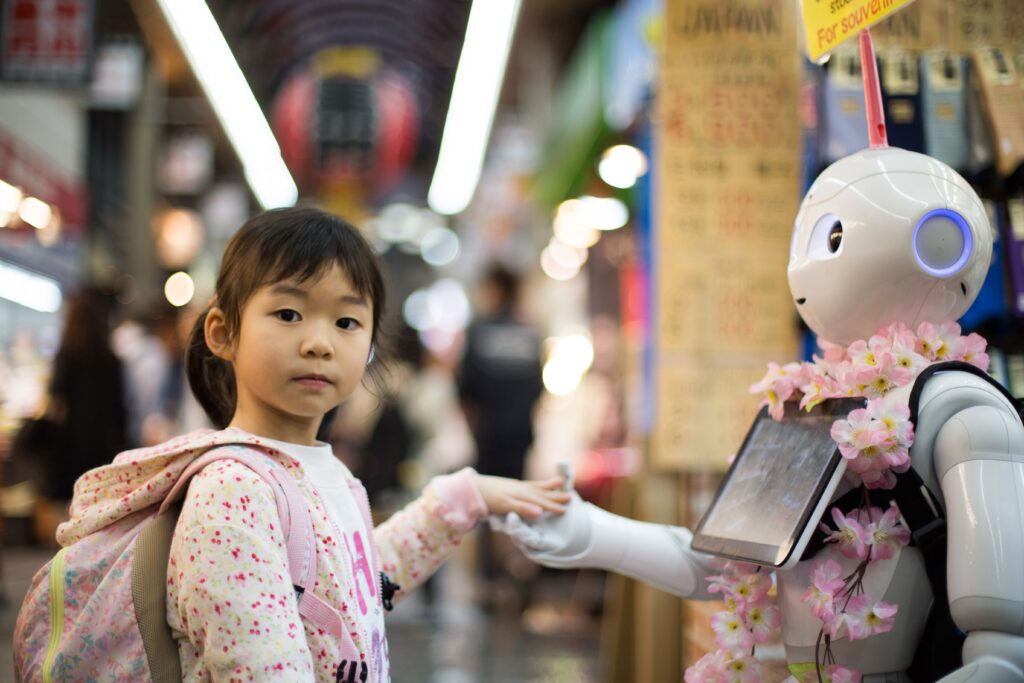
We’d all love to have a smart robot in our house that understands spoken natural language, just like those in sci-fi films. How far are we from this dream?
An anonymous author once mused that “AI is the science of making computers act like the ones in the movies”. Assuming they meant something like the computer on the starship Enterprise or Rosie, the household robot from “The Jetsons”, what would be required to achieve this dream? And where do we stand?
A modest version of a household robot should be able to understand and respond to simple spoken instructions in natural language (any language spoken by people, such as English, Spanish or Chinese). It should be able to run errands and perform basic chores, and its responses should be reasonable. That is, we can’t expect robots to be correct all the time, but in order to be trustworthy, a robot’s responses should make sense.
For our household robot to react reasonably to requests such as “get the blue mug on the table”, it should be able to deal with several issues, such as perceptual homonymy (words that mean different things under different perceptual conditions), syntactic ambiguity, and user vagueness and inaccuracy. It should also be able to recognise users’ intentions, the potential risks of actions and adapt to different users.
Perceptual homonymy applies to intrinsic features of objects, such as colour and size, and to spatial relations. For example, when talking about a red flower or about a person’s red hair, the two colours are usually completely different. In other cases, the intended colour may be hard to determine, or an object could have several colours.
Size depends on the type of an object. For instance, a particular mug may be considered large in comparison to mugs in general, but it is usually smaller than a small vase. In addition, context matters: objects seem smaller when placed in large spaces, and if there are two mugs on a table — a larger one and a smaller one — and a user requests a large mug, our robot should retrieve the former.
Spatial relations can be divided into topological relations (indicated by prepositions such as “on” and “in”) and projective relations (signalled by prepositional phrases such as “in front of” and “to the left of”).
Looking at topological relations, “the note on the fridge” may be vertically on top of the fridge or attached to the front of the fridge with a magnet. Also, if we ask our household robot for “the apple in the bowl”, an apple sitting inside a fruit bowl would satisfy this requirement, but so would an apple on top of a pile of apples in a bowl (even if this apple exceeds the height of the bowl), because it is within the control of the bowl (if we move the bowl, the apple will move with it). However, if an apple was glued to the outside of the bowl, it would still be within the control of the bowl, but we wouldn’t say it is in the bowl.
Projective relations depend on a frame of reference, which may be the speaker, the robot or a landmark. For example, if we ask our household robot to pick up the plant to the left of the table, do we mean our left or its left? A similar decision would be made when interpreting “the plant in front of the table”, but not for “the plant in front of the mirror”, as a mirror has a “face” (it only has one front).
These problems are exacerbated by errors in Automated Speech Recognition — the technology that allows people to speak to computers. Automated Speech Recognition errors may happen due to out-of-vocabulary words or rare words, which a speech recogniser may mishear as a common word, or words that are being used outside their usual context. Table 1 illustrates three errors made by a speech recogniser for the description “the flour on the table”.

Our AI should be able to cope with misheard and out-of-vocabulary words. For instance, if we request “the shiny blue mug”, and our robot can’t identify shiny objects, it should still be able to generate a useful response, such as “I can’t see ‘shiny’, but there are two blue mugs on the table, which one do you want?”. Eventually, our robot should be able to learn the meaning of some out-of-vocabulary words.
The robot will also have to contend with syntactic ambiguity, vagueness and inaccuracy. Syntactic ambiguity occurs when the phrasing of a description licenses several spatial relations. For instance, if we ask for “the flower on the table near the lamp”, who should be near the lamp? The flower or the table? A request for “the blue mug on the table” is vague when there are several blue mugs on the table, and inaccurate when the mug on the table is green, or the blue mug is on a chair.
Having some concept of a speaker’s intention, and of the implications of requested actions, would help our robot respond appropriately. If we are thirsty, then even if our request is ambiguous or inaccurate, the robot could bring one of several mugs. But this is not the case if we want to show our special mug to a friend. What if we ask the robot to throw a chair? When would it be appropriate for our robot to question our request, and when should it just comply? An implicit assumption made by optimisation-based response generation systems is that there is one optimal response for each dialogue state. However, our response-generation experiments have shown that different users prefer different responses under the same circumstances, and that several responses are acceptable to the same user. Therefore, it is worth investigating user-related factors, such as habits, preferences and capabilities, which influence the suitability of an AI’s responses.
Moving forward, in order to generate suitable responses to a user’s request, an AI should be designed with the ability to assess how good its favourite candidate interpretation is, how many other good candidates there are, and how they differ from this favourite interpretation.
To achieve that, our AI would have to keep track of alternative interpretations; and for each interpretation, the AI would compute the probability that it was intended by the speaker and the utility associated with it. This probability, in turn, would incorporate the probabilities of the following factors: the output of the speech recogniser, the syntactic and semantic structures of the user’s request, and the pragmatic aspects of the interpretation.
Previous work has offered a computational model that implements this idea with respect to descriptions comprising simple colours, sizes and spatial relations. To reach a desirable endpoint, this approach would have to be extended to consider the more complicated issues raised above. Designed correctly, AIs of the future should consider all these factors to determine whether its interpretations make sense; and they should be able to discern between several plausible interpretations, and decide when to ask and when to act.
Professor Ingrid Zukerman works in the Department of Data Science and Artificial Intelligence in the Faculty of Information Technology. Her areas of research are explainable AI, dialogue systems, trust in devices, and assistive systems for elderly and disabled people.
The research on which this article is based was funded in part by the Australian Research Council.
Professor Zukerman extends many thanks to Wendy, Ashley and Debbie Zukerman for their helpful comments during the preparation of this article.
Originally published under Creative Commons by 360info™.